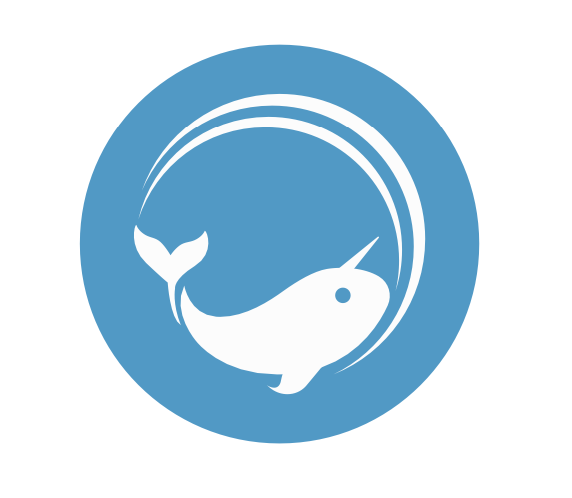
Vini AI
Medical history collection, interpretation and diagnosis using LLMs, embeddings, Vector+RDBMS for large scale deployments
Why combine AI approaches?
Large language models (LLMs) like chatgpt excel at understanding meanings in language, but they usually falter when factual information is required (they generate hallucinations, generic answers, etc).
LLMs are also very expensive in terms of computational resources. These translate in high costs and worse: high latencies (response times) for most of the interesting use cases. A strategy based only on LLMs does not scale to high volume applications like PGD for prevention or follow-up.
AI is not only LLMs, and there is much to be gained by combining other techniques that have greater speed and higher accuracy at the expense of needing a more structured approach.
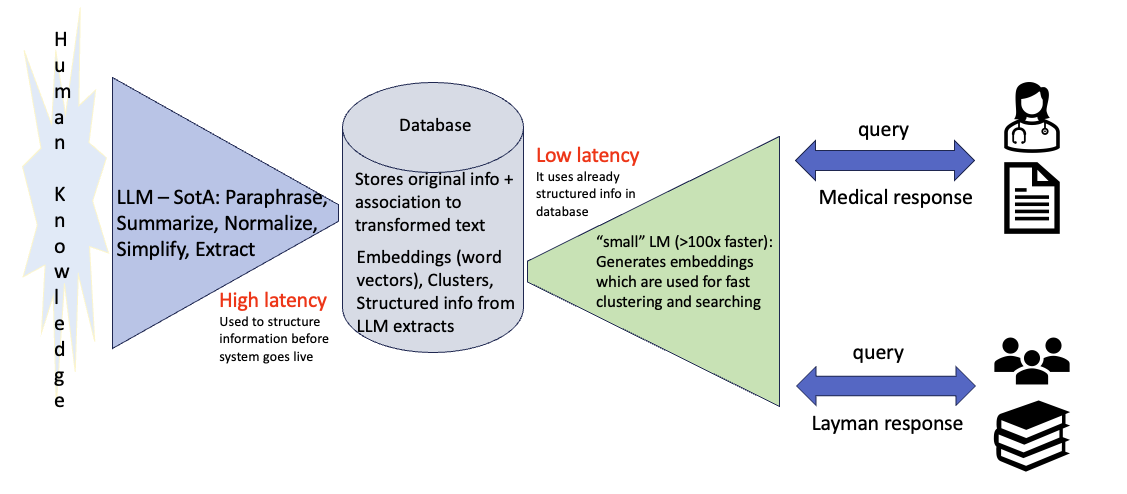
Use LLMs to structure information
Use LLMs where latency and high cost have little impact: backend to structure information, let reliable and swifter techniques handle the front-end.
Example - WHO ICD-11
A database compiled by the World Health Organization that lists over 18,000 medical conditions in more than 20 categories.
Since the audience is Medical practitioners the classification and language are very technical.
We use an LLM (like Mistral, Llama, Gemini, etc) to transform this information into a database with layman descriptions and questions.
Technical medical to layman
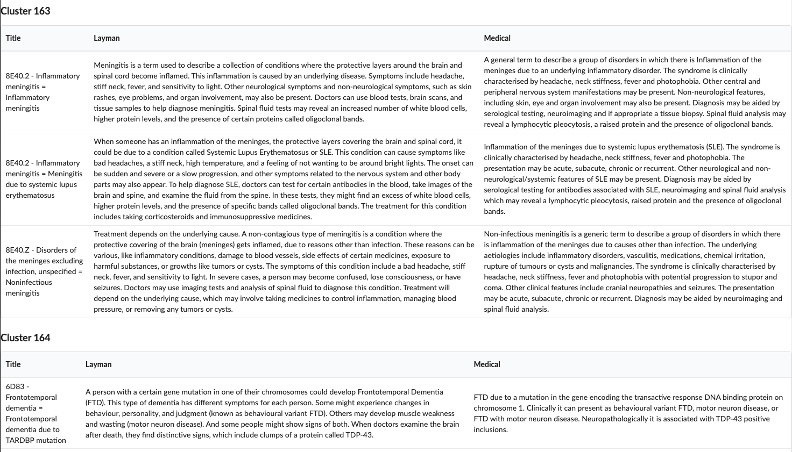
Generate questions and de-duplicate
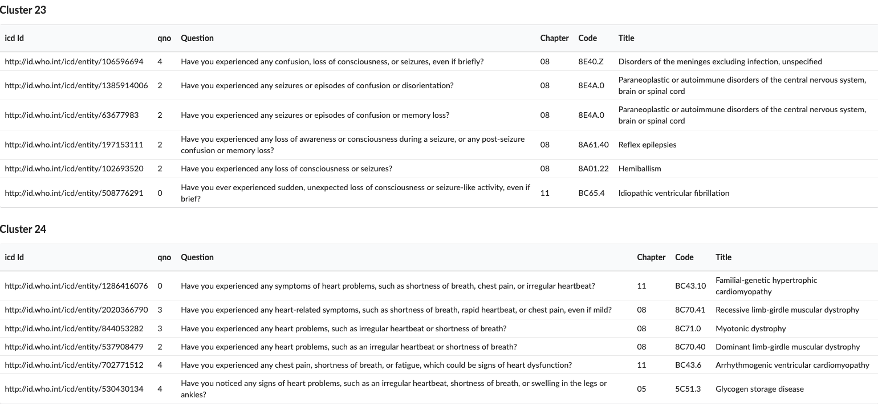
Questionnaire for general public
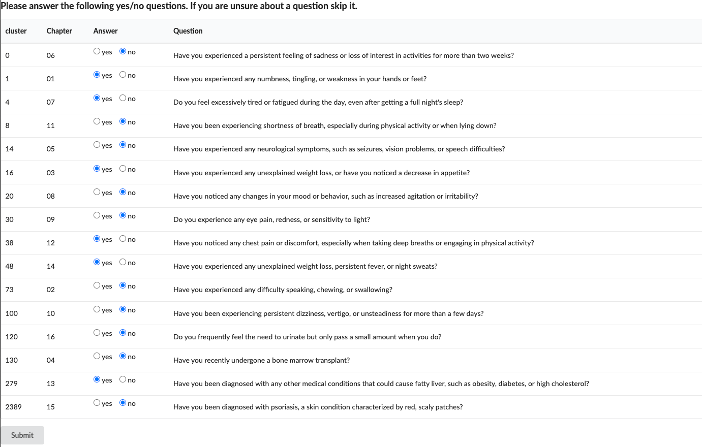
Pre-diagnostic for Medical personnel
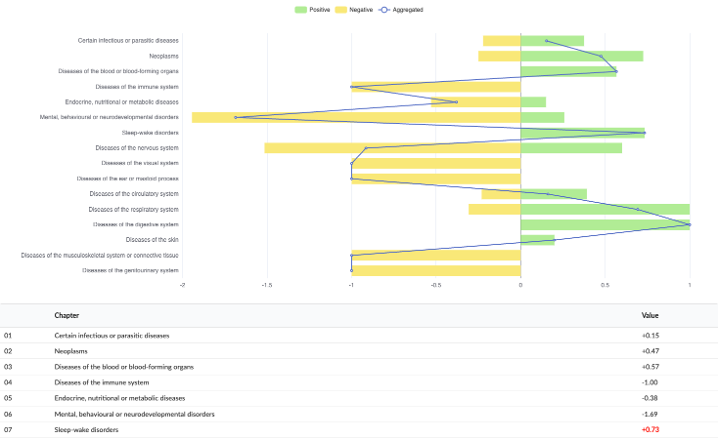
The TLDR;
By combining different LLM sizes and capabilities plus “older” machine learning techniques like clustering and vector search using embeddings we can get the best of all worlds by investing a little engineering effort.
Information already processed and stored in the database can also be used to generate information sites about the different afflictions for different audiences. By associating diagnostic to ICD coding we can help EHR and follow-up with treatment and/or medical wellness therapies.
This information workflow can be applied to any other knowledge area where interaction between experts and laypeople happens.